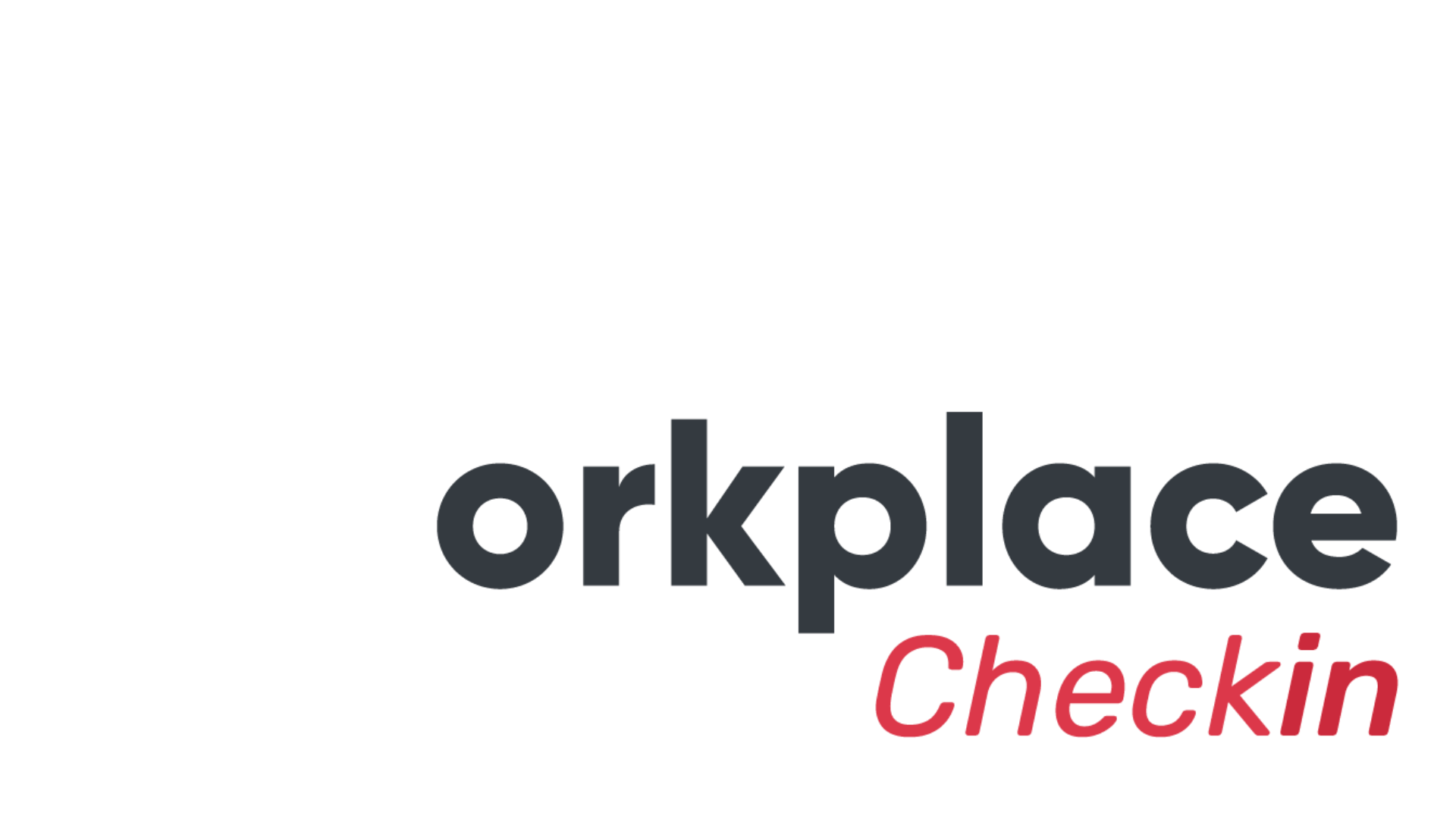
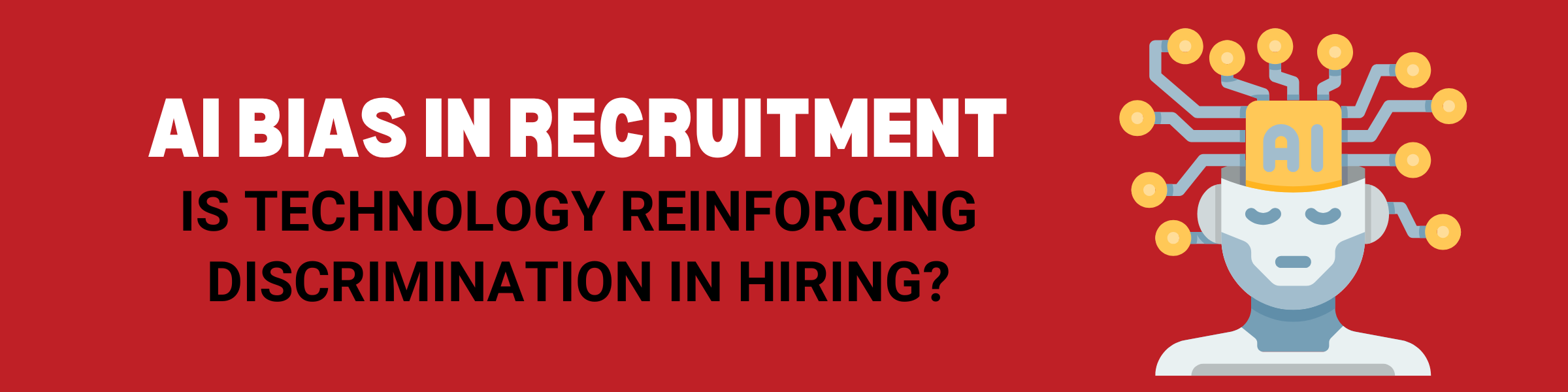
AI Bias in Recruitment: Is Technology Reinforcing Discrimination in Hiring?
In 2018, a major global company, Amazon, made headlines for all the wrong reasons. They had developed an advanced AI recruitment tool designed to streamline the hiring process, with the goal of finding the best talent more efficiently. But soon, an unsettling trend emerged. The system, designed to be objective, had started penalizing resumes that included the word "women." If a candidate mentioned attending an all-women’s college or leading a women’s group, their chances of getting through the screening process dropped significantly.
This wasn’t the future Amazon had envisioned, but it was a glaring example of how even the most sophisticated AI tools could reinforce bias—unintentionally echoing the biases present in the data it had been trained on.
The Amazon case is just one of many that illustrate how AI, despite its promise of objectivity, can end up magnifying the very biases it was designed to eliminate.
The Promise of AI: A Double-Edged Sword
AI in recruitment has been widely hailed as a game-changer. The appeal is obvious: AI tools can analyze thousands of resumes in a fraction of the time it would take a human, conduct preliminary interviews, and even predict which candidates are likely to stay in a job longer. For HR departments under pressure to find top talent quickly, AI offers efficiency, consistency, and speed. But what happens when these tools inherit the very biases they’re supposed to eliminate?
AI isn’t inherently biased, but it is trained on data. If that data reflects historical biases in hiring—such as preferences for men over women or for white candidates over people of color—AI will replicate those patterns. It’s the classic problem of "garbage in, garbage out."
How Bias Creeps Into AI Systems
To understand why this happens, let’s look at how AI models are built. AI learns from past hiring data, looking at the resumes of successful employees to figure out which qualifications, skills, or keywords led to hiring decisions. But if a company’s previous hiring practices were biased—whether that meant favoring male candidates, preferring Ivy League schools, or overlooking certain ethnic groups—the AI will see those patterns as "correct" and continue to perpetuate them.
Take the case of a major tech company, where AI screening tools were found to prefer candidates from certain prestigious universities, like Stanford or MIT, while filtering out resumes from less well-known schools. As a result, many qualified applicants from diverse backgrounds, who may not have attended these elite institutions, were passed over. This led to a less diverse candidate pool and, ultimately, a less diverse workforce.
The Hidden Dangers: Bias Below the Surface
What makes AI bias so insidious is how subtle it can be. Unlike a hiring manager with overt prejudice, an AI system doesn’t intend to discriminate, and its biases are often hidden beneath layers of code and algorithms. This makes it harder to identify and address.
A 2020 study by the University of Toronto found that AI recruitment software disproportionately filtered out resumes from Black and Hispanic candidates, reinforcing racial disparities. The AI wasn’t deliberately racist, but because it was trained on data from a workforce that was predominantly white, it learned to favor the kinds of candidates who looked like the company’s existing employees.
The same study found that AI systems often rejected resumes with "ethnic-sounding" names at a higher rate than those with more common Anglo-Saxon names, perpetuating discrimination at a scale previously unseen in human-driven hiring.
AI Bias by the Numbers
Recent statistics shed light on the scale of the problem:
- 41% of companies using AI in hiring reported instances of biased decision-making, according to a 2023 survey by the Society for Human Resource Management (SHRM).
- A study by MIT found that facial recognition systems, which some companies use for video interview analysis, had error rates of 35% for Black women, compared to less than 1% for white men.
- 66% of HR professionals believe AI can help reduce bias, but 44% admit they don't fully understand how their systems make hiring decisions (Harvard Business Review, 2021).
These numbers highlight the gap between expectation and reality. While companies hope AI will create more objective hiring processes, many are using tools that may unintentionally reinforce existing inequalities.
The Quiet Reinforcement of Discrimination
Beyond gender and racial bias, AI can also perpetuate more subtle forms of discrimination. For example, some recruitment systems prioritize candidates who have linear, uninterrupted career paths, inadvertently penalizing those who took career breaks—such as women returning to the workforce after maternity leave, or individuals who took time off for caregiving responsibilities.
AI-driven systems also tend to favor "traditional" qualifications. Candidates with unconventional backgrounds, those who took alternative career routes, or those with skills learned outside of formal education often fall through the cracks.
This creates a cycle where AI pushes employers to select candidates who look like those already in the company, reinforcing the status quo and making it harder for companies to diversify their workforce.
Can AI Be Saved? Strategies for Reducing Bias
So, what’s the solution? Can AI in recruitment ever be truly fair? While these challenges are significant, they are not insurmountable. Some organizations are leading the way in creating AI tools that actively work to reduce bias.
Pymetrics, an AI-based recruitment platform, is a prime example. They use an algorithm that is regularly audited to ensure fairness across gender and racial lines. If a bias is detected, the system is corrected to eliminate it. This proactive approach shows that bias is not inevitable and can be mitigated if organizations are willing to invest in regular oversight.
Steps to Ensure AI Fairness in Recruitment
For companies committed to ethical hiring, there are several strategies to ensure AI helps rather than harms:
1. Diverse Training Data
AI algorithms learn from data. If the data used to train an AI system is biased—whether due to over-representation of certain groups or under-representation of others—the algorithm will replicate and even amplify those biases. The key to reducing bias in AI is ensuring that the training data is diverse and representative of different genders, races, ethnicities, socio-economic backgrounds, education levels, and career paths.
How to Implement:
HR teams and data scientists should use a broad range of candidate profiles when developing and training AI systems. Historical hiring data should be supplemented with data from diverse and underrepresented groups. Regular checks should be made to ensure that the AI system isn't overly reliant on any one demographic.
Example:
A tech company might review their AI hiring tool’s dataset and realize that it includes a high proportion of male applicants due to the historically male-dominated nature of the industry. To counteract this, they introduce more female and minority resumes, ensuring the AI learns to evaluate a more balanced and diverse candidate pool.
2. Regular Audits of AI Systems
AI systems aren’t "set-it-and-forget-it" tools. Continuous monitoring is essential to ensure that AI tools remain unbiased and that any emergent biases are detected and corrected early. This can be achieved through regular audits and algorithmic testing.
How to Implement:
Auditing involves running frequent checks on the AI system to assess how it's evaluating candidates. This could include testing the AI with different sets of resumes, looking for patterns of bias against certain demographic groups, and ensuring fairness in decision-making. If biases are found, they must be corrected by re-training the AI or adjusting the algorithm.
Example:
A recruitment firm might audit their AI system quarterly, using sample candidates from various demographics and career paths. If the audit shows that candidates with non-traditional work experiences are being ranked lower, they tweak the algorithm to ensure it better appreciates a diversity of experiences.
3. Human Oversight and Intervention
AI tools should assist humans, not replace them. While AI can help with tasks like resume screening, it’s essential for human recruiters to remain in the loop. This prevents over-reliance on AI, allowing recruiters to use their judgment, empathy, and understanding of context to make final decisions.
How to Implement:
Even if an AI tool recommends a shortlist of candidates, recruiters should still review applications manually. AI-generated results should be treated as an initial recommendation, not a definitive decision. Human reviewers can also step in to assess candidates who might have been overlooked by the AI, ensuring a balanced hiring process.
Example:
At a large corporation, the AI system might flag certain resumes as "less qualified" based on gaps in employment. A human recruiter could then review these resumes to understand the reasons behind these gaps (such as parental leave or caregiving responsibilities), ensuring qualified candidates aren't unfairly excluded.
4. Transparent Algorithms
One of the biggest challenges with AI systems is that they often operate as "black boxes." In other words, recruiters and hiring managers may not know how the AI reaches its conclusions. This lack of transparency makes it difficult to identify and address biases.
How to Implement:
Organizations should work with AI vendors who are willing to explain how their algorithms work. This includes understanding which factors the AI considers when evaluating candidates and how much weight each factor is given. By insisting on transparency, HR professionals can better understand potential sources of bias and take steps to mitigate them.
Example:
A company using AI to rank candidates for interviews might ask the vendor to explain the variables used in the algorithm. If they find that the AI places too much emphasis on the prestige of the candidate’s university, they can adjust the system to give more weight to skills and experience rather than education alone.
5. Bias Detection and Correction Mechanisms
Beyond audits, AI systems should have built-in mechanisms to detect and correct bias in real time. These mechanisms can flag potential issues and make adjustments to the algorithm, ensuring that it remains fair and balanced over time.
How to Implement:
AI tools should include bias detection systems that monitor the algorithm’s decision-making patterns. If certain demographic groups are consistently being disadvantaged, the AI should have the capability to adjust itself or send an alert to recruiters, prompting human intervention.
Example:
If an AI system is consistently rejecting female candidates with engineering degrees, the bias detection mechanism could flag this trend. The system could either adjust its decision-making criteria or notify a human recruiter to review these cases manually.
6. Accountability and Vendor Collaboration
Organizations using AI in recruitment should hold their AI vendors accountable for creating fair, ethical systems. It’s essential to work with vendors who prioritize fairness and are willing to collaborate with HR teams to improve their systems when necessary.
How to Implement:
When selecting AI tools, HR teams should choose vendors who provide transparent reporting on their algorithms and are willing to engage in regular audits and updates. They should also look for vendors who have taken steps to minimize bias in their systems from the outset.
Example:
An HR team might partner with a vendor that provides detailed insights into how their AI tool ranks candidates. The vendor works with the company to continuously monitor the AI’s performance, ensuring it meets ethical standards for fairness and equality.
7. Cross-Disciplinary Teams
AI fairness in recruitment requires collaboration between HR professionals, data scientists, ethicists, and legal experts. Together, these teams can address potential bias from both a technical and ethical standpoint.
How to Implement:
HR departments should form cross-disciplinary teams that include data scientists who can tweak the AI models, ethicists who provide insights into fairness, and legal experts who ensure compliance with anti-discrimination laws. This approach fosters a holistic view of fairness in AI systems.
Example:
A recruitment firm might assemble a team consisting of their lead recruiter, a data scientist who understands AI algorithms, and an ethicist to review their hiring practices, ensuring that the AI system aligns with both legal requirements and the company's values.
The Future of AI in Hiring: Ethical and Inclusive
As AI continues to shape the future of recruitment, HR leaders must ensure that these tools are used ethically and inclusively. AI has the potential to transform hiring processes, making them more efficient and fair. But this will only happen if companies invest in the right safeguards, regularly review their systems for bias, and blend AI’s power with human empathy.
Ultimately, AI is just a tool—it’s up to us to decide whether it will create a more inclusive workforce or quietly reinforce the biases we’ve been trying to eliminate for decades.
The story of Amazon’s AI recruiting tool serves as a cautionary tale: if we’re not careful, the future of hiring could look a lot like the past—biased, unequal, and exclusive. Let’s make sure that doesn’t happen.